How Focus Attributes improve comparability of interview scorecards

Comparison is fundamental to hiring. Companies must compare the qualifications and experience of a candidate to the requirements of a role, as well as to the qualifications of other candidates and this starts with interview scorecards. Gathering the information necessary to make these comparisons often requires multiple interviewers and interviews, as well as "micro-comparisons" along the way, such as determining which candidates to advance for further interviews.
It’s vital for companies to maintain consistency when interviewing to ensure they’re making fair comparisons across candidates – apples to apples, if you will. They should collect the same relevant data from each candidate at the same point in the process, as this creates an equitable experience for candidates and ensures the company is making fair and informed decisions.
To illustrate why this is important, suppose a company is looking to add a new member to their accounting team. They’ve identified ten criteria that are important, and after interviewing two candidates, they have the following information:
How should the company decide which candidate to advance?
One candidate seems more knowledgeable on SaaS metrics, but the company doesn’t know how competent they are with GAAP accounting. The other candidate knows GAAP well, but there’s no information about their communication skills. If both candidates were interviewed by the same person, perhaps the interviewer could fill in the missing information. However, there’s a chance they might misremember or unconsciously use irrelevant information that biases their reassessment, such as a choppy internet connection or finding out they shared a major in school (which introduces similar-to-me bias). If each candidate had a different interviewer, filling in the gaps becomes harder and more prone to bias. The problem compounds further as the number of candidates and interviewers increases.
Structured interviewing helps companies avoid this scenario by maintaining consistency in how they collect the information they need to make effective and fair hiring decisions. Research shows that asking all candidates the same question in a specific interview and rating their individual responses can reduce bias and make it easier to compare candidates on individual job requirements (Campion et al. 1997, Bohnet 2016). This also improves the candidate experience by minimizing the likelihood of repeat questions throughout the interview process.
Greenhouse uses the concept of "scorecard attributes" to represent the specific job criteria customers use to evaluate candidates. After each interview, interviewers submit scorecards rating individual attributes. These attributes allow for visual and easy-to-understand comparisons between candidates and also give the hiring team the ability to determine whether a candidate meets specific job criteria. To make sure data is consistent, Greenhouse also implements a feature called "Focus Attributes,'' which draws interviewer attention to the specific attributes they are supposed to rate.
After a group of candidates has gone through a set of interviews, hiring teams will often compare them and determine who to advance. It is important to collect the same information from each candidate. This ensures that the comparisons are effective at identifying the strongest candidate(s) and fair to everyone who participated in the interviews.
To assess whether Focus Attributes facilitate this process, we need to determine the comparability of interview scorecards. Dice Similarity, a statistic used to gauge the similarity of two samples, is a suitable measure for this. In our case, Dice Similarity calculates similarity based on how frequently attributes are rated between two interview scorecards versus how often they are not, excluding cases where attributes are not rated on either scorecard. The score falls between 0 and 1, with higher values indicating more similarity. Below, we give examples of pairs of interview scorecards, along with their corresponding Dice Similarity score, illustrating how more consistent data collection translates to a higher score.


By calculating the Dice Similarity between all unique pairs of scorecards on each interview and taking the average, we can estimate the consistency of data collection on that interview. A higher average indicates that interview scorecards are more comparable to one another.
To understand the effect of Focus Attributes on comparability, we combine these scores with other information about the interview. For example, we know how many attributes a job has, and whether a particular interview uses Focus Attributes. We also know which attributes were rated on a given scorecard, and how many interviewers were involved. Using data from jobs created in 2022, we evaluate the comparability of approximately 10.3 million interview scorecards on approximately 1.28 million interviews.
Our analysis suggests that configuring Focus Attributes leads to a substantial increase in average Dice Similarity, estimating that comparability is 31 - 38% higher when an interview uses Focus Attributes.
By controlling for other interview design factors, it’s also possible to see how job and interview configuration affect comparability. For example, we see that comparability tends to be lower when jobs have many attributes. Fortunately, we also see that interviews with Focus Attributes are still more comparable when the number of attributes is very high. However, this trend suggests that companies should be deliberate about the attributes they evaluate, and not create more than they need.
Similarly, the number of Focus Attributes in an interview can lead to lower comparability if there are too many assigned. It’s important to strike a balance between collecting data efficiently and not overwhelming interviewers. Based on the trends in our analysis, assigning three to five Focus Attributes in an interview appears to strike a good balance between maximizing data collection and candidate comparability.
Focus Attributes can be especially helpful in cases where an interview requires many interviewers or many scorecards. Comparability drops when going from one interviewer to two, and that trend continues downward when more interviewers are added. However, the average remains higher on interviews with Focus Attributes; further, the average appears to stabilize and may actually increase. Similarity also decreases when interview scorecard volume is high, but interviews with Focus Attributes still tend to have higher similarity among scorecards than interviews without them, regardless of scorecard volume. This may speak to the ability of Focus Attributes to maintain alignment across larger interviewer pools and extended hiring processes.
Though this analysis focused on the overall effectiveness of Focus Attributes on improving comparability, we are also interested in how this may affect candidates from different demographic groups. We know from our research on anonymizing take-home tests that applicants from historically underrepresented racial groups face a disadvantage when take-home tests are not anonymized. In future research, we plan to investigate whether data collection is less consistent for candidates from different demographic groups, what effect that has on interview pass rates and whether configuring Focus Attributes contributes to those outcomes.
Overall, Focus Attributes are an effective way to increase consistency in data collection among interviewers by directing them to the specific attributes they need to evaluate. This improves the validity of decisions about specific candidates and comparisons between candidates. By ensuring that candidates are comparable to one another at each stage of the interview, each candidate has a fair opportunity to showcase their qualifications. This also allows the company to make an informed decision on which candidates to move forward.
Focus Attributes can be particularly helpful when many different interviewers will be assigned the same interview. It is still important to be mindful of the number of attributes on a job overall, as well as the number of attributes assigned on specific interviews, as assigning too many can overwhelm the interviewer and decrease consistency. Overall, Focus Attributes are a relatively simple way to embed interview instructions in the interview user interface (UI) and improve candidate comparability.
Interested in learning more about best practices when using interview scorecards? Read this guidance article.
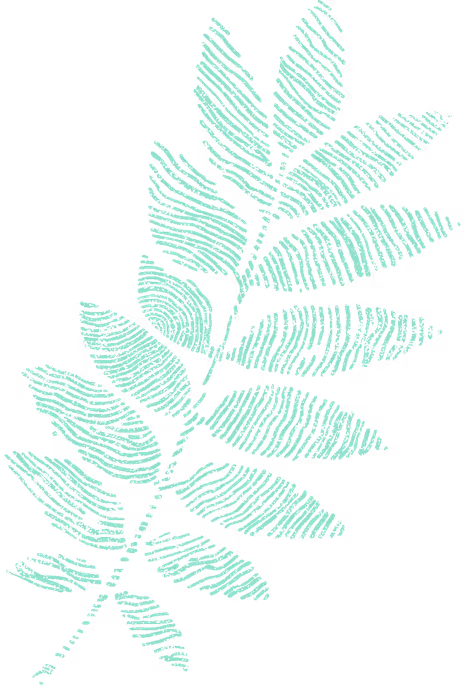