How anonymous take home tests mitigate bias and improve candidate odds
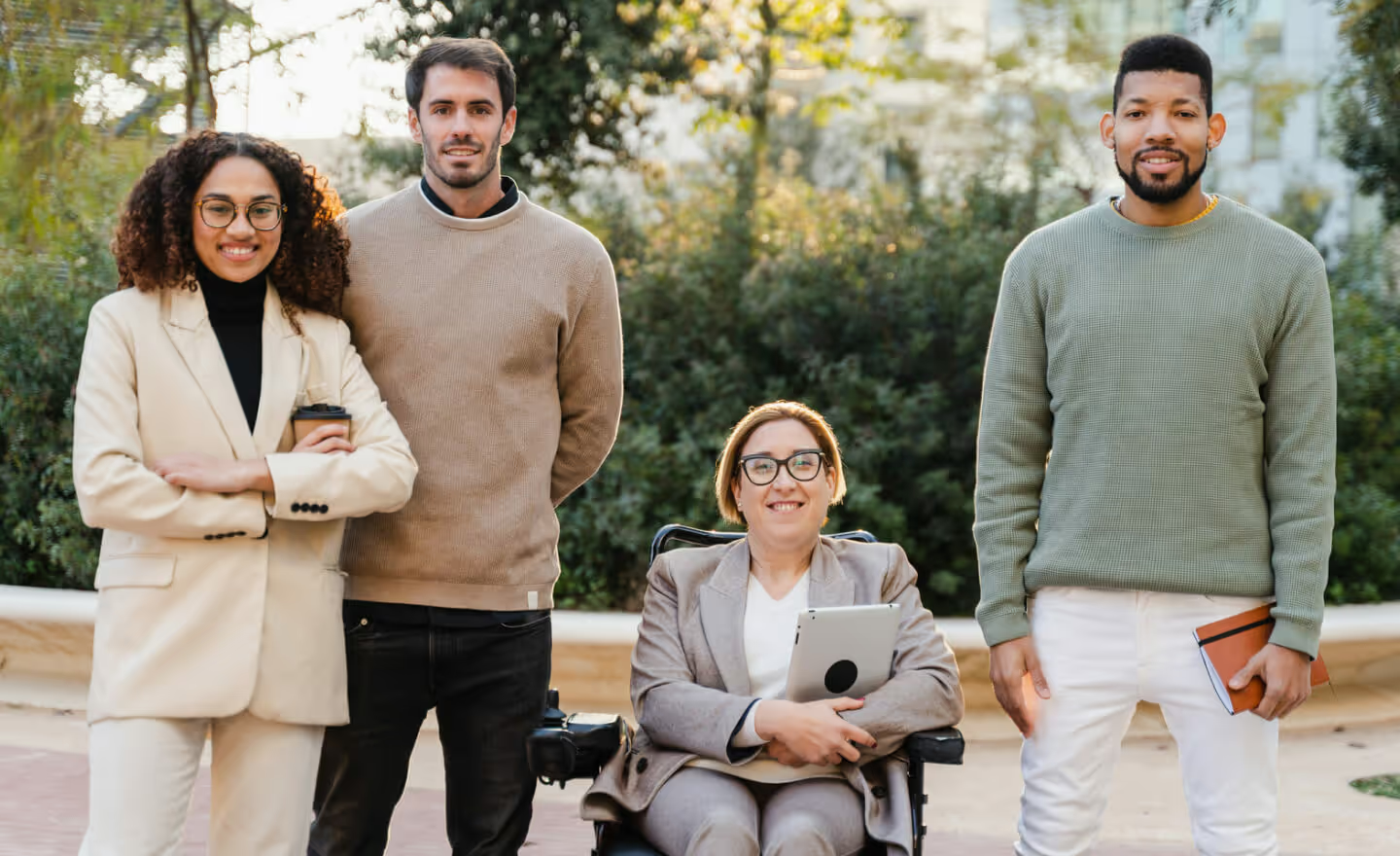
When it comes to hiring, there’s a large body of research demonstrating how irrelevant factors can influence hiring decisions. These could be general cognitive biases, such as the halo effect or favoring someone who shares a hobby, but there can also be bias towards candidates of a certain age, race or gender that affects who gets hired. In all cases, these biases— which are often unconscious— reduce our ability to focus on useful information in our decision-making, leading to poorer hiring decisions.
To mitigate bias, many companies ask candidates to complete a take home exercise to evaluate them on skills necessary for the job. By focusing on the skills necessary for the role rather than the candidate’s pedigree, companies are better suited to identify strong candidates. While take home tests can lessen bias, they do not necessarily remove bias by themselves.
Within Greenhouse, customers can utilize our Take Home Test feature in a job’s interview plan to configure an assessment. This allows them to send an exercise to applicants and receive the completed exercise, where an assigned grader then evaluates the test. Our Expert-tier customers can also anonymize a Take Home Test during grading. This prohibits the grader from seeing the candidate’s profile or identifying information from the test, preventing them from doing additional research on the candidate that could bias their evaluation.
Figure 2: Example of an anonymized Take Home Test
What does anonymized grading reveal about non-anonymized test bias?
We can use aggregated data from our product to assess whether a candidate becomes more or less likely to pass a Take Home Test when grading is anonymous. For customers that collect EEOC (Equal Employment Opportunity Commission) data, we can also see what demographic biases may be at play when grading is not anonymous.
With a dataset of approximately 384,000 applicants who submitted a Take Home Test between January 2021 and December 2022 on jobs collecting EEOC data, we conducted regression analyses to estimate the likelihood that a candidate passes an interview. We considered the following variables: grading method used (anonymous or not), overall recommendation a candidate received (grouped as positive or negative), role type (e.g. Engineering roles, roles with multiple hires) and candidates responses to race and gender EEOC questions.
Our results indicate that anonymization provides a general benefit to all candidates: we estimate the pass-through rate for a Take Home Test increases by 6.5-10% when grading is anonymous. When a scorecard is submitted, we estimate an additional increase of 6-13% — even in cases where the scorecard is negative. This suggests that the cumulative increase is anywhere from 12-23%.
When we consider EEOC data, we see that applicants from historically underrepresented groups are less likely to pass relative to White applicants when grading is not anonymized: Black candidates are 7.4-12.4% less likely to pass and Hispanic candidates are 2-7.5% less likely. These negative effects are often offset or erased when grading is anonymized. This illustrates the bias at play for Black and Hispanic individuals, and how anonymization can mitigate that bias.
Predicted likelihood of passing a Take Home Test, based on Overall Rating, Race and Test Anonymization. Predictions are based on a Linear Probability Model including all job openings. Note that we only include results for applicants who self-identified as Asian, Black or African American, Hispanic or Latino, or White on the EEOC questionnaire because those were the Race variables where we had statistically significant coefficients.
Pass through rates observed in the dataset, broken out by Overall Rating, Race and Test Anonymization. Note that we only include results for applicants who self-identified as Asian, Black or African American, Hispanic or Latino, or White on the EEOC questionnaire because those were the Race variables where we had statistically significant coefficients.
Similarly, when looking at gender we saw that female candidates are 2-3% less likely to pass than male candidates when grading is not anonymous. Anonymized grading improves the chances of a female candidate passing the interview, but unfortunately, our results indicate that the increase in chance of passing is still less than that of male applicants.
This result persisted in our intersectional analysis: to the extent anonymizing a Take Home Test helped offset an initial negative bias towards a particular race (e.g. towards Hispanic applicants), the offset primarily benefited male applicants within that race. Overall, female applicants are still more likely to pass if grading is anonymous, but the pass-through rates between men and women grow further apart.
Predicted likelihood of passing a Take Home Test, based on Overall Rating, Gender and Test Anonymization. Predictions are based on a Linear Probability Model including all job openings.
Pass through rates observed in the dataset, broken out by Overall Rating, Gender and Test Anonymization.
The results suggest that structural aspects of take home exercises can disadvantage certain groups, particularly female applicants.
For example, asking candidates to spend a certain number of hours on a Take Home Test may disadvantage candidates with less free time. Candidates with more free time also may choose to ignore the time limit, putting others at a disadvantage. Though we could not examine this question more specifically in our analysis, we think it’s important for companies to consider how the requirements they choose to include on a Take Home Test may disproportionately disadvantage candidates from particular demographic groups.
Our evidence suggests that most applicants will have a better chance of passing a Take Home Test when grading is anonymized, indicating a tendency for people to base their decision-making on information outside of what is relevant to the test when it’s available. Anonymized grading helps mitigate this tendency, elevates candidates’ ability to showcase their skills and improves a company’s chance of identifying people with the talents they seek.
Visit our support page to learn more about the Take Home Test feature and how to get started in Greenhouse.